Nursing is a rapidly growing area worldwide, with outputs both in practice and research. With the focus on evidence-based practice, which emphasises critical thinking and using the best available evidence, the ways in which nurses acquire evidence need to be considered. Research is one of the most important ways of obtaining evidence to inform practice. In principle, good research is expensive, with ethical considerations and requirements, and a need for relevant education and training (Jolley, 2020); increased nursing research funding means more research programmes and output, contributing to high-quality, safe practice. Attributes acquired in research, such as critical thinking, are also important characteristics for successful nursing practice (Ingham-Broomfield, 2014; Liu et al, 2019).
Nurse researchers can use a variety of methods, whether qualitative, quantitative or mixed. The choice of method will depend on the individual project, and how best to address the research questions/hypotheses (Duffy, 1985; Simonovich, 2017; Leedy and Ormrod, 2020). In the early years, nursing research mainly used quantitative approaches, but there has been a shift towards the use of qualitative methods (Driessnack et al, 2007). Quantitative methods, either as stand-alone research or as part of a mixed-methods approach, require knowledge of mathematics (especially statistics) because they require data collection, manipulation in a numerical form and analysis using relevant software (for example, the SPSS statistical software package). This process is essential in quantitative research, allowing nurse researchers to investigate phenomena and present their findings in a clear and concise way (Babbie, 2020).
There do appear to be problems with quantitative research in nursing. Nurses themselves, although recognising statistics as an important element in nursing research, appear unhappy with the level of their own statistical knowledge and abilities (Gaudet et al, 2014; Jones et al, 2021). Studies have revealed similar results with identified issues in the statistical capabilities of nursing faculty members (Hayat et al, 2021) and student nurses (Kiekkas et al, 2015; Chiesi and Bruno, 2021). Some authors have identified errors in the methodology and analysis of results in published quantitative studies in the field of nursing (Anthony, 1996; Staggs, 2019; Hayat et al, 2021; Jones et al, 2021; Schroeder et al, 2022).
Although Jones et al (2021) found that many nurse researchers struggle with statistical analysis, Hayat et al (2021) suggested that targeted educational interventions could significantly improve these skills. However, there is still a lack of consensus on the most effective training methods. For nurse researchers to be confident and comfortable in using statistics in research, they need to be familiarised with these early in their nursing career. It is important that they start this familiarisation during their undergraduate education and continue to receive ‘refreshers’ and updates tailored to their needs and level of education and training. This kind of support throughout their studentship or professional career would have a significant impact (Taylor and Muncer, 2000; Kiekkas et al, 2015; Jones et al, 2021).
Presenting appropriate statistical tests for nursing research in appropriate outlets (such as peer-reviewed journals) could enhance access and familiarisation for the wider nursing research community. Besides choosing publication outlets to which nurses have better access, the presentation of methods applicable to nursing research and the use of nursing-specific examples will also contribute to readers’ understanding. With the use of a nursing-specific example, terminology is placed within readers’ familiar frame, which simplifies the related concepts (Benner, 1984). The hope is that with this familiarisation, there will be fewer mistakes in the methods and results sections of quantitative nursing publications.
This article, in line with this approach, aims to offer guidance and support to research nurses by presenting a widely used statistical test, the independent samples t test (Box 1).
Steps in determining reliability using SPSS software (Cronbach's alpha)
Inferential statistics
When using the independent samples t test, the aim is to investigate if there is a difference between the means of two samples that are independent (Heavey, 2022). With the application of this t test, researchers aim to determine if the results are statistically significant. That is, they aim to distinguish between results among their samples that arose by chance, and results that can be generalised and represent a whole population. The closer to 0% the probability for error is, the more the results are accepted as reproducible and can be reported as generalised to the total population (Taylor and Muncer, 2000; Staggs, 2019; Heavey, 2022).
In social sciences, the acceptable probability for error is usually set to a maximum of 5%. This 5% is the agreed chance for the researcher to be mistakenly rejecting the null hypothesis. Keeping this acceptable threshold in mind, results smaller than 0.05 are reported as ‘statistically significant’. However, to be able to generalise to the total population, nurse researchers need to also be able to support their statistically significant results with a relevant large effect size. Large effect size means that results are also practically significant and can indeed be reported as such.
To use the independent samples t test successfully, nurse researchers need to be clear about certain characteristics of their samples (Heavey, 2022):
If the data do not follow a normal distribution but rather a skewed one, and contain ordinal data (with ranking among them), then a similar but different test is suggested, the Mann Whitney U test.
The use of a relevant statistical software package (such as SPSS, JMP, Minitab, etc) can be a great help in the statistical process. It can run all calculations in the background and provide users with the test outputs. In brief, the process in conducting the test entails the data collection, the data entry into the statistical software package (eg SPSS), the check for normality assumptions of data, the performance of the test and the production of results that will be analysed (Figure 1).

Reliability check
Before getting into the detailed actions of the independent samples t test, internal consistency (reliability) of the used items needs to be checked and reported (Chatzi and Doody, 2023). For survey research, one suggested way is to look for questionnaires that have already been used with acceptable reliability scores. This will also help produce results comparable with past research (Chatzi and Doody, 2023). It is important to measure internal consistency, as it reveals whether acceptable scores for items (questions) of similar characteristics can be reproduced (Tavakol and Dennick, 2011).
Cronbach's alpha (α) is a reliability coefficient that provides a method of measuring internal consistency of tests and is the most common measure used for this purpose. Cronbach's alpha can be calculated for items that are already clustered from past studies or are clustered in the study at hand due to the similarity of their topics (responses coding should be similar as well). The steps for Cronbach's alpha calculation in SPSS can be found in Box 1. Alpha coefficient results greater than or equal to (≥) 0.7 indicate reliable results and therefore are considered acceptable for the research project (Tavakol and Dennick, 2011). However, for small groups of items (consisting of two to four items) Cronbach's alpha might produce smaller results. For such groups it is suggested the researcher use the less biased Spearman-Brown coefficient (Eisinga et al, 2013). When Cronbach's alpha/Spearman-Brown values are calculated and found acceptable, then the researcher needs to create a new variable of mean values for each of the clusters where a Cronbach's alpha/Spearman-Brown test has been run with acceptable results. These variables of means will then be used for the independent samples t tests.
Independent samples t test
Suppose a gynaecology ward nurse researcher is called to investigate whether there is a difference in female patients' systolic blood pressure measurements between those who have had hysterectomy surgery and women who have had urinary incontinence surgery. The study involves 200 female patients, with 100 having undergone hysterectomy surgery and 100 having had urinary incontinence surgery. Data on systolic blood pressure measurements are to be collected using standardised medical equipment within 24 hours following surgery. To proceed with this project, the nurse will use the independent samples t test to compare the means of systolic blood pressure measurements of the two groups of patients. When comparing means, the independent samples t test results will either support or reject the null hypothesis (H0=there is no difference in systolic blood pressure measurements). In the case that the t test results are significantly different (P≤0.05) between the groups, the alternative hypothesis (H1=there is difference in systolic blood pressure measurements) is accepted instead. The alternative hypothesis implies that the examined groups appear to have significantly different means. For this example, the systolic blood pressures of the two groups of patients are different, with hysterectomy surgery patients observed to have higher systolic blood pressures than urinary incontinency surgery patients.
The next step is to conduct the independent samples t test for the two means of systolic blood pressure measurements of the two independent groups of patients. Box 2 outlines the steps to follow when using SPSS. After performing the test, the software will report results in three tables:‘Group Statistics’, ‘Independent Samples Test’ and ‘Independent Samples Effect Sizes’, as shown in Figure 2.
Steps in running the independent samples t test using SPSS software
Independent samples t-test
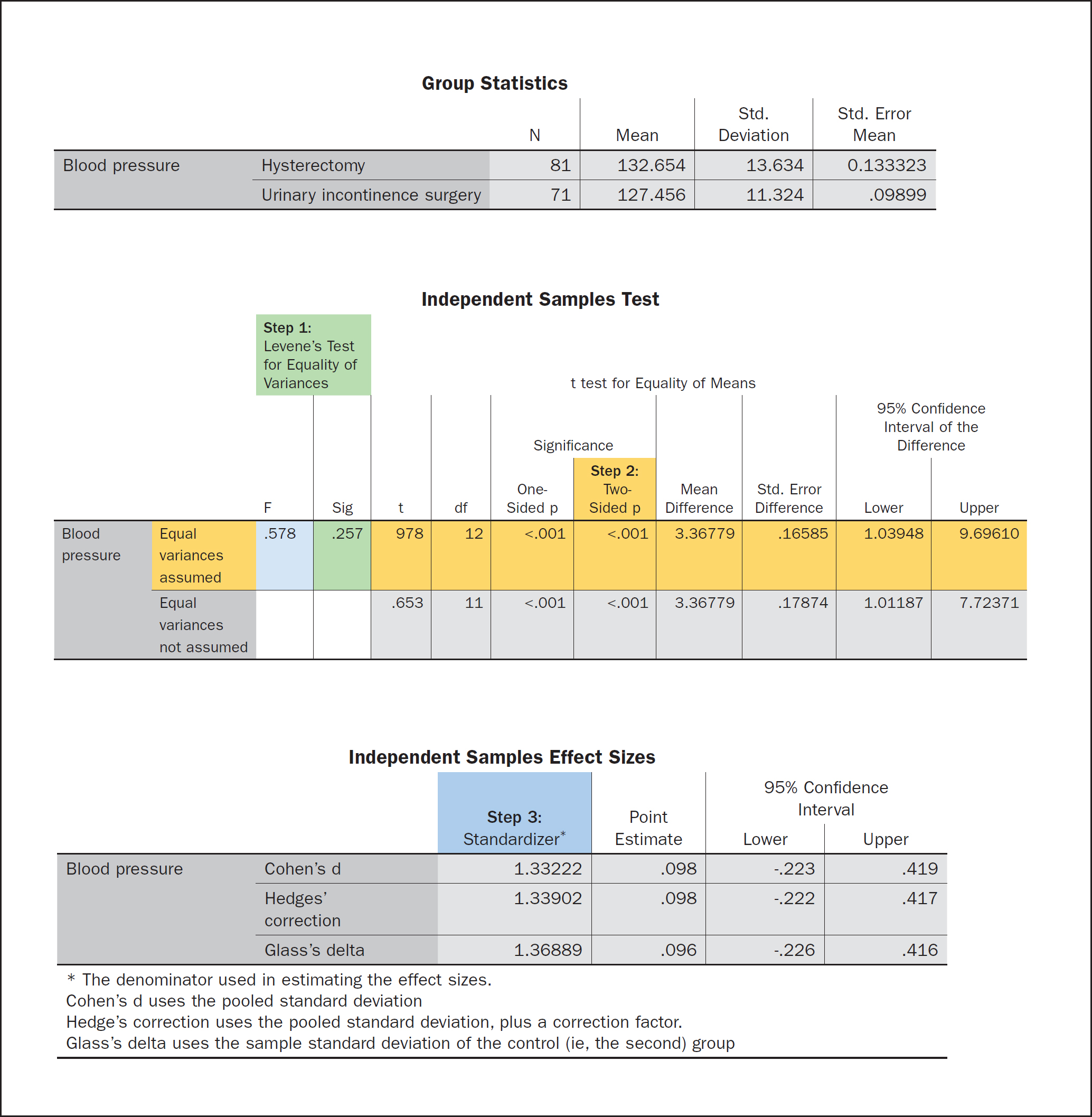
Variance
The first step (step 1 in green, Figure 2) is to assess the results of Levene's test for equality of variances in the table named ‘Independent Samples Test’ to determine whether equal variances are assumed or not assumed (test for homogeneity of variance). SPSS runs Levene's test to compare the two groups of patients for the same variance. A note needs to be made here that Levene's P value results are quite sensitive to the sample size. With big sample sizes, Levene's P value tends to be smaller, and the opposite is noted with small sample sizes. For this reason, while assessing the Levene's P value, considering the sample size and effect size (the actual variances and the differences between them) will help the researcher in avoiding over-stating results (with large samples) or under-stating results (with small samples). In the relevant table, the results of Levene's test are shown in the column marked ‘Sig.’
Assessing Levene's test for equality of variances is important as it determines which line of results in the table (‘Equal variances assumed’ or ‘Equal variances not assumed’) to read for the independent samples t test results. In this exemplar case, for a significance result (Sig) of 0.257, which is greater than 0.05, the null hypothesis is not rejected, and equal variances are assumed, therefore the first line of results is followed in the table (step 2 in yellow, Figure 2). If the significance result in Levene's test for equality of variances were less than 0.05, the null hypothesis would be rejected, and equal variances would not be assumed, therefore, in the SPSS table, the second line of results would be followed. Again, it should be noted here that even though the SPSS software labels the two options as ‘Equal variances assumed’ and ‘Equal variances not assumed’, it runs more underlying tests in the background. These tests (adjustment to the degrees of freedom (df) using the Welch-Satterthwaite method) help produce the correct results when homogeneity assumptions (Levene's test) are not met (Laerd Statistics, 2018).
Moving back to the exemplar case, the next step would be to read the line (in the same table) of the t test results for equality of means. In this line, the important results to focus on are the t values and P values. For the P values, one must look at the ‘Significance, Two-Sided p’ column of the table (Figure 2). This is the result of the two-tailed test, which is the one most frequently used as it allows the researcher to explore the difference of the two means in all directions (for the example here, systolic blood pressure measurements could be higher or lower). Therefore, in the systolic blood pressure project, the ‘Two-Sided p’ (P value) result on the first line (‘Equal variances assumed’) (Figure 2) is the one that will be used to determine the project's independent samples t test result. The P value is <0.001 (which is less than 0.05), therefore the null hypothesis is rejected, and the researcher could suggest that there is statistical difference in the average systolic blood pressure between hysterectomy surgery and urinary incontinence surgery patients.
Effect size
However, P values are not enough to determine the significance of results. Calculating the effect size and power will provide more evidence in the reporting of results. This additional calculation will assist in determining whether statistically significant results are also practically significant. The reporting of both P values and effect size and power (step 3 in blue, Figure 2) will support further the generalisation of results to the wider population and their usefulness for evidence-based nursing practice.
Even when power has been considered when defining adequate sample size at the outset of a project, sample errors can not be entirely avoided. As sample errors need to be addressed, the calculation of effect size is a very important step. An effect size ‘expresses the average magnitude of an intervention's effect as a standard deviation; thus it offers an indicator of the usefulness of the intervention’ (Taylor and Muncer, 2000; Shin, 2009). An adequate large effect size supports the assumption that results are clinically significant and can be projected to the whole population, or generalised (Taylor and Muncer, 2000). For this reason, calculating and reporting effect size is strongly recommended (Fritz et al, 2012).
When running the independent samples t test using SPSS, the relevant output is included in a table labelled ‘Independent Samples Effect Sizes’ (Figure 2). Cohen's d values can be used to report effect sizes for differences between two groups of samples (Lovakov and Agadullina, 2021). Cohen's d is deemed appropriate for big group sizes, whereas for very small groups (<20), Hedge's g is recommended instead. Hedge's g indicates the effect size of the difference in means (how much one group differs from another group) due to the large difference in sample sizes. For both Cohen's d or Hedges' g, a score of 0.20 suggests small effect size, 0.50 medium effect size, and 0.80 large effect size (Lovakov and Agadullina, 2021). When results come back showing a large effect size, this suggests research findings with practical significance, whereas a small effect size corresponds to limited practical applications. The whole process of the independent samples t test, with all included tests, is presented graphically in Figure 3.
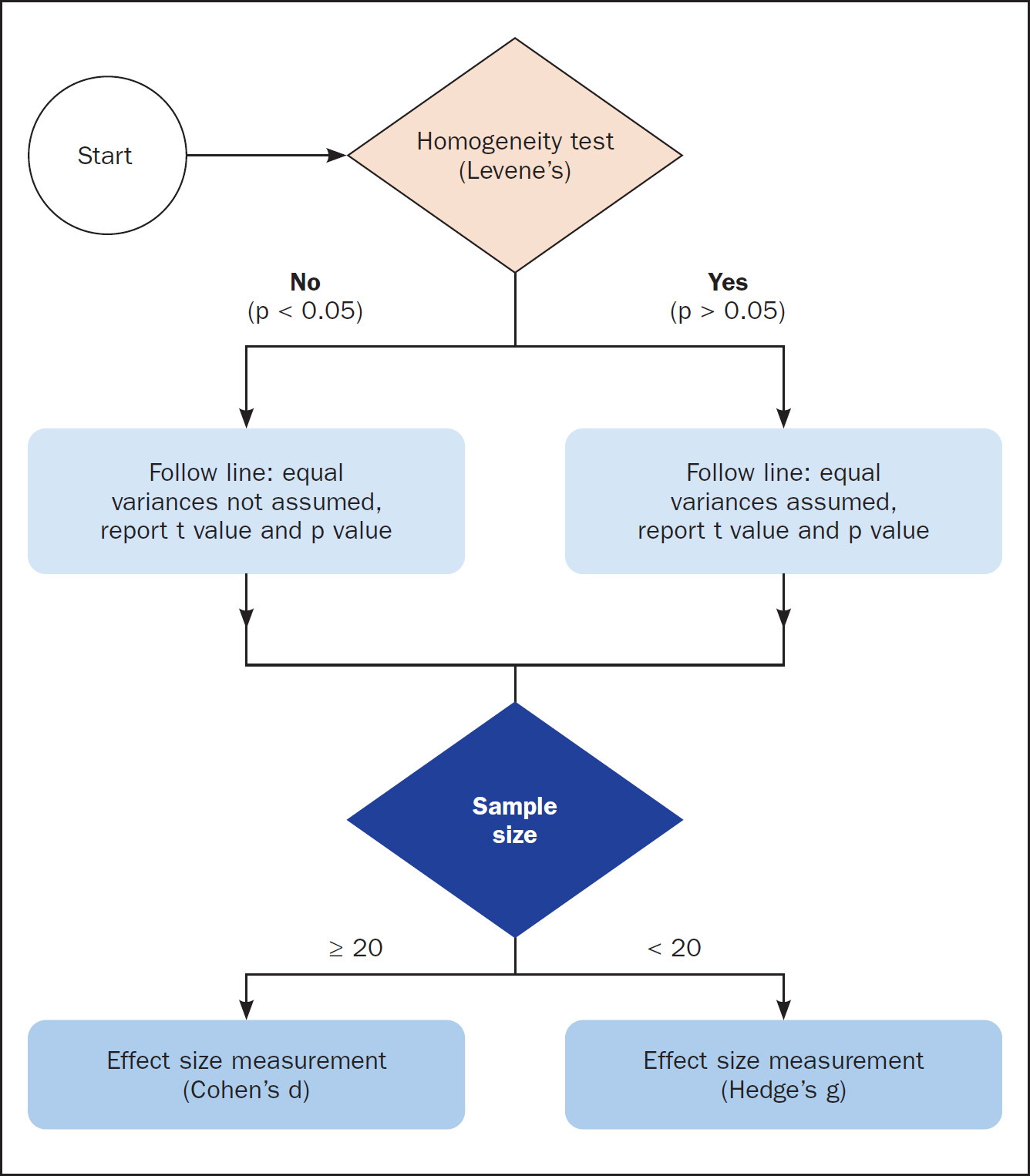
Reporting and interpreting results
To start drafting a project report or journal article manuscript, there are certain basic rules to follow for cohesion and precision. In the ‘results’ section any descriptive results are presented first, including Cronbach's alpha results. The inclusion of all these results is important, as they provide evidence of appropriate methodology selection for the research questions/hypotheses (Leedy and Ormrod, 2020).
Next, in seeking to test the research questions/hypotheses, one by one the relevant results are presented. For a comprehensive presentation of results, at least two outputs are proposed: a graph of the means of the two variables and a table with their number (n), mean, standard deviation, t value, df, P value and effect size (Cohen's d or Hedge's g). The effect size results add weight to the t test's results, as they add to the clinical significance of results and assist the researcher in determining whether they can be generalised to the wider population and research literature. Levene's test results are not usually reported, they are used by the researcher for the identification of the independent samples t test correct results. Critical discussion of results and comparisons with past research are mostly handled in the ‘discussion’ section of the report or manuscript.
Regarding the wording of the results and discussion sections, reporting clinically significant results follows careful assessment of the independent samples t test results in conjunction with the effect size. This will help draw more informed conclusions on the population - which is the purpose of inferential statistics (Taylor and Muncer, 2000; Shin, 2009). Another important issue that needs to be highlighted here is that statistical analysis is conducted to detect important effects. Therefore, strong expressions such as ‘prove’ or ‘substantiate’ are not recommended. Researchers are instead advised to use less emphatic terms such as ‘appear’, ‘show’, ‘suggest’, ‘interpret’, ‘imply’. As an example for the presentation of the exemplar results here, the following sentence is proposed: The significant difference in systolic blood pressure between hysterectomy surgery and urinary incontinence surgery patients suggests that postoperative care protocols may need to be adjusted to address higher risks of hypertension in hysterectomy surgery patients. Future research should explore tailored interventions to mitigate this risk.
Conclusion
Evidence-based practice is the way for nursing to continue to evolve. Nursing research is one of the most important ways to obtain this evidence, yet quantitative research has been shown to be sometimes problematic for nurse researchers. There is a need for enhanced statistical training in nursing education, such as regular workshops on statistical methods in nursing curricula, and ongoing professional development courses or outlets to ensure nurse researchers are well-equipped to conduct robust quantitative studies.
This article has attempted to provide nurse researchers with the complete process and understanding of successful implementation of an independent samples t test, through a nursing-specific example, to simplify the quantitative concepts. As well as understanding the necessary steps for performing the tests with statistical software, it is important to report results and findings in a cohesive and robust manner.